How AI & ML are Revolutionizing Product Quality Control
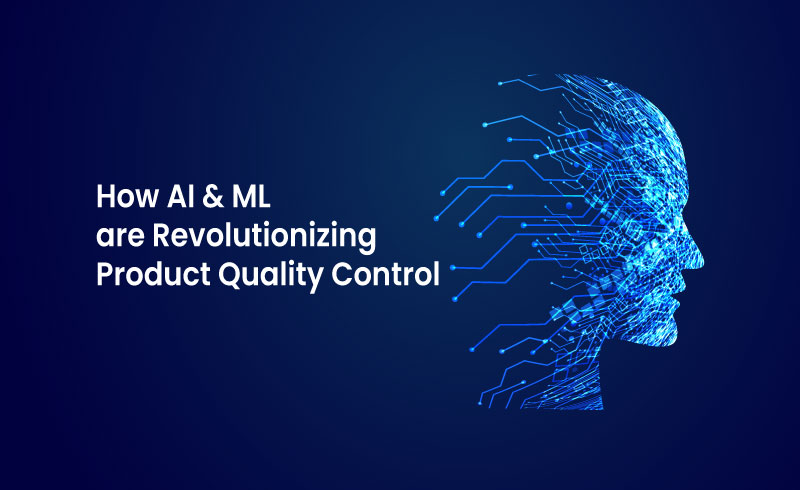
Introduction
Artificial Intelligence (AI) and Machine Learning (ML) are changing the way product quality control is done. They offer unmatched accuracy and efficiency, making traditional methods of quality assurance that heavily rely on human expertise and manual inspection outdated.
AI automates many parts of the quality control process, such as assessing product criteria and spotting defects in real-time. This automation not only makes inspections faster but also reduces the chances of human mistakes. By being able to quickly analyze large amounts of data, even the tiniest flaws can be found, greatly improving overall product quality.
ML techniques take this a step further by continuously learning from new information, leading to better defect detection over time. Algorithms can be trained to identify patterns and irregularities that human inspectors might overlook. This adaptive learning feature makes machine learning extremely valuable in maintaining high standards during production.
Benefits and Impact
- Increased Efficiency: Automation speeds up the quality control process.
- Enhanced Accuracy: Real-time defect identification reduces human error.
- Continuous Improvement: ML algorithms evolve with new data inputs.
By incorporating AI and ML into quality control processes, businesses can not only improve their performance but also gain valuable insights for ongoing enhancements. These technologies enable companies to meet strict production standards while minimizing expenses and satisfying customers better.
Automating Quality Control Processes with AI
AI technology is revolutionizing product quality control by automating various processes that traditionally relied heavily on human intervention. Automation in quality control involves using sophisticated AI algorithms to perform tasks such as inspection, testing, and defect detection, significantly increasing efficiency and accuracy.
How AI Technology is Used to Automate Quality Control Processes
AI technology leverages advanced computer vision and machine learning algorithms to scrutinize products at various stages of the production line. For instance:
- Visual Inspection: AI-driven systems use high-resolution cameras paired with image recognition software to assess products for defects that might be missed by the human eye.
- Functional Testing: Automated systems can simulate usage conditions to ensure product functionality meets predefined standards.
- Dimensional Accuracy: Laser scanning and other precision measurement techniques are employed by AI systems to verify the exact dimensions of products, ensuring they adhere to specifications.
Advantages of Utilizing AI for Product Quality Control
Implementing AI in product quality control offers several distinct advantages:
- Consistency: Unlike humans, AI systems do not experience fatigue or variation in performance, resulting in consistent and reliable quality assessments.
- Speed: Automated inspections are faster than manual processes, allowing for real-time evaluation and quicker decision-making.
- Cost Efficiency: Reducing the need for extensive human labor and minimizing errors leads to significant cost savings.
- Scalability: AI systems can easily scale up operations to handle increased production volumes without compromising on quality.
Real-time Defect Identification and Resolution with AI
One of the most impactful benefits of using AI in quality control is its ability to identify and resolve defects in real-time. By continuously monitoring the production process, AI systems can:
- Spot anomalies instantly, reducing the likelihood of defective products reaching customers.
- Provide immediate feedback to manufacturing equipment, enabling on-the-fly adjustments that correct issues before they propagate further down the line.
- Gather data on defect patterns, which can be analyzed to pinpoint root causes and prevent future occurrences.
Utilizing real-time defect identification ensures that products meet high standards consistently while also maintaining efficient production workflows. This proactive approach not only enhances product quality but also boosts overall operational efficiency.
By integrating AI into your quality control processes, you harness a powerful tool capable of transforming how defects are detected and resolved. This sets a solid foundation for exploring even more advanced techniques such as machine learning enhancements.
Enhancing Quality Control Through Machine Learning Techniques
Machine learning (ML) techniques are transforming quality control by significantly enhancing both the accuracy and speed of defect detection. By leveraging ML algorithms, businesses can move beyond traditional inspection methods, which often rely heavily on human intervention and are subject to errors.
Key Benefits of ML in Quality Control:
- Enhanced Accuracy: ML algorithms analyze vast amounts of data to identify patterns and anomalies that may indicate defects. This leads to more precise identification of issues compared to manual inspection.
- Increased Speed: Automated ML systems process information faster than human inspectors, allowing for real-time monitoring and quicker responses to defects.
- Scalability: As production scales up, ML systems adapt easily, maintaining consistent quality checks without the need for proportional increases in human resources.
Improving Defect Detection with Machine Learning Algorithms
ML algorithms continuously learn from new data, enhancing their defect detection capabilities over time. Here’s how they contribute:
- Pattern Recognition: ML models excel at recognizing complex patterns within datasets. This capability is particularly useful in identifying subtle defects that might be missed by traditional methods.
- Anomaly Detection: By setting baseline standards, ML algorithms can detect deviations from these norms, flagging potential defects early in the production process.
- Predictive Maintenance: ML techniques predict when equipment might fail or produce substandard products, enabling preemptive maintenance actions to avoid costly downtimes.
Real-World Applications
Several industries have successfully integrated ML into their quality control processes:
- Automotive Manufacturing: Companies use ML to inspect car parts for defects automatically, ensuring each component meets stringent safety standards.
- Electronics: In semiconductor manufacturing, ML algorithms detect minute imperfections in circuits that could affect performance.
- Pharmaceuticals: Ensuring the purity and consistency of drugs is critical; ML aids in identifying contaminants and variations in drug formulations.
By integrating machine learning techniques into quality control processes, businesses achieve higher accuracy and efficiency, leading to improved product reliability and customer satisfaction.
The Power of AI, ML, and Human Expertise in Quality Assurance
To achieve the best results in quality assurance, it’s important to combine the strengths of AI & ML technologies with human involvement. While AI and ML can automate many parts of quality control, human experts bring a level of understanding and decision-making skills that are essential.
How AI, ML, and Human Expertise Work Together for Better Quality Assurance
Here’s how the synergy between AI, ML, and human expertise enhances quality assurance:
Leveraging Human Insight and Experience
- Humans have deep contextual knowledge and can make complex judgments that machines may struggle with.
- Their expertise is crucial for interpreting data generated by AI systems and making informed decisions.
Harnessing the Power of AI for Insights
- AI excels at processing large amounts of data quickly, identifying patterns, and providing insights that human inspectors might miss.
- These insights can guide human experts to focus on critical areas that require attention.
The Role of AI and ML in Improving Quality Control Processes
AI-powered insights can be instrumental in driving process improvement within quality control:
Making Data-Driven Decisions
- AI algorithms analyze production data to identify inefficiencies or recurring defects.
- This enables continuous improvement in manufacturing processes.
Predicting Equipment Maintenance Needs
- By predicting when equipment is likely to fail, AI systems help schedule maintenance at optimal times.
- This reduces downtime and prevents defects from occurring.
Ensuring Real-Time Monitoring
- Continuous monitoring powered by AI ensures that quality standards are maintained throughout the production process.
- This allows for immediate adjustments to be made if necessary.
How Machine Learning Enhances Human Capabilities in Quality Assurance
Machine learning algorithms play a crucial role in enhancing human capabilities within quality assurance:
Offering Actionable Insights
- Through advanced analytics, ML provides actionable recommendations to improve product quality.
Supporting Training and Development
- ML models can be used to simulate different scenarios, aiding in the training and development of human inspectors.
Facilitating Collaboration
- Integrating AI/ML with collaborative platforms allows teams to share insights and best practices.
- This fosters a culture of continuous improvement within the organization.
By leveraging the strengths of both advanced technologies and human expertise, businesses can achieve unparalleled levels of quality assurance. This synergy not only ensures high-quality products but also drives innovation and efficiency in production processes.
Predictive Analytics, Root Cause Analysis, and Beyond: Advanced Techniques Enabled by AI/ML in Quality Control
Predictive analytics and root cause analysis are game-changers in quality control, driven by AI and ML technologies. These advanced techniques enable businesses to anticipate potential issues and address them proactively, transforming traditional quality control approaches.
Predictive Maintenance
AI/ML systems excel at predictive maintenance by analyzing historical data to forecast potential equipment failures. This capability helps organizations:
- Minimize downtime
- Extend the lifespan of machinery
- Reduce maintenance costs
Machine learning algorithms identify patterns in data that humans might overlook. By predicting when a component is likely to fail, companies can schedule maintenance activities at optimal times, ensuring continuous production without unexpected interruptions.
Facilitating Root Cause Analysis
Identifying the root cause of defects is crucial for maintaining high-quality standards. AI/ML technologies streamline this process by:
- Analyzing vast amounts of data from various sources
- Detecting anomalies and correlations
- Providing actionable insights
These systems can pinpoint the exact cause of a defect more accurately than traditional methods. The insights gained enable teams to implement effective corrective actions, preventing recurrence and improving overall product quality.
Leveraging Advanced Analytics Capabilities
Advanced analytics powered by AI/ML offer several benefits:
- Real-time monitoring: Continuous tracking of production processes to detect deviations immediately.
- Data-driven decision making: Utilizing detailed analytics reports to guide strategic decisions.
- Scalability: Easily scaling quality control measures across multiple production lines or facilities.
By leveraging these capabilities, organizations achieve a higher level of precision in their quality control processes. The integration of predictive analytics and root cause analysis not only enhances efficiency but also ensures consistent product quality.
Incorporating these advanced techniques enables businesses to stay competitive in an increasingly demanding market. The synergy between AI/ML technologies and traditional quality control methods creates a robust framework for achieving excellence in product quality assurance.
Challenges and Future Implications of Adopting AI and ML in Product Quality Control
Adopting AI and ML for product quality control brings with it several challenges that require careful consideration.
Implementation and Management Challenges
Here are some key challenges that organizations may face when implementing and managing AI/ML systems for quality control:
- Data Quality and Quantity: AI/ML systems need high-quality, extensive datasets to train effectively. Poor data can result in inaccurate predictions and faulty quality assessments.
- Integration with Legacy Systems: Many industries still rely on traditional quality control systems. Integrating modern AI/ML technologies with these legacy systems can be complicated and expensive.
- Skill Gap: Successfully deploying AI/ML in quality control requires specialized knowledge. However, the current workforce may lack the necessary skills, which means companies will need to invest in training or hiring new talent.
- Cost Considerations: The initial setup costs for AI/ML systems can be significant, including expenses for hardware, software, and human resources. This might make it difficult for small to medium-sized businesses to afford implementing these technologies.
Future Implications
There are several trends that we anticipate will shape the future of AI and ML in quality assurance:
- Increased Adoption of Edge Computing: To reduce delays and improve processing speeds, more companies are likely to embrace edge computing. This shift will enable real-time quality control on a larger scale.
- AI-Driven Predictive Maintenance: By using AI to predict equipment failures before they happen, organizations can minimize downtime and enhance overall efficiency.
- Enhanced Human-Machine Collaboration: The collaboration between human expertise and AI capabilities will continue to evolve. Humans will increasingly rely on AI insights for decision-making while focusing on tasks that require human creativity and judgment.
- Regulatory Compliance: As the use of AI/ML systems becomes more widespread, regulatory bodies may introduce new guidelines for their implementation in quality control. It will be crucial for businesses adopting these technologies to stay compliant with these regulations.
Navigating these challenges while leveraging emerging trends will determine the successful integration of AI and ML into product quality control processes. Companies that are prepared to adapt will position themselves as leaders in innovation within the field of quality assurance.
Conclusion
Exploring the potential of AI & ML in transforming your quality control processes can bring significant improvements in efficiency and accuracy. By integrating these advanced technologies, you can elevate your quality assurance measures, ensuring higher standards and enhanced customer satisfaction.
Engaging with these materials will provide you with a comprehensive understanding of how AI and ML can revolutionize your approach to product quality control. Leveraging these technologies not only keeps you ahead in the industry but also ensures that your products meet the highest standards of excellence.