How is AI Changing Healthcare Diagnosis and Treatment?
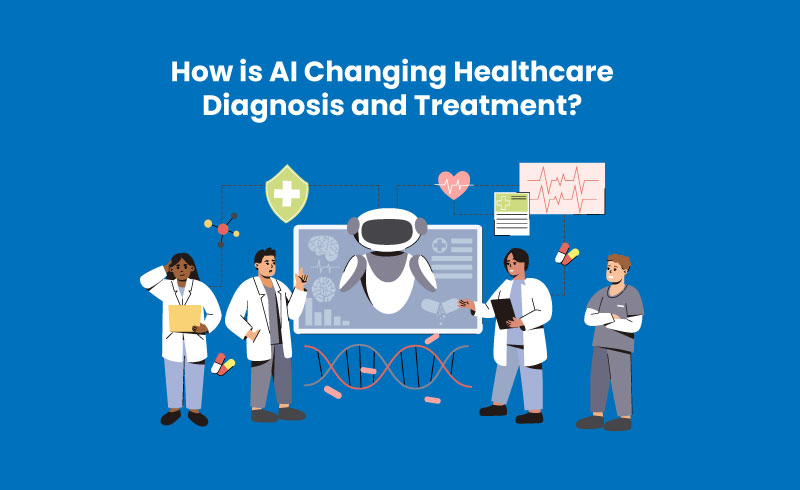
Artificial Intelligence (AI) is increasingly playing a transformative role in healthcare, particularly in the areas of diagnosis and treatment. By leveraging sophisticated algorithms and vast datasets, AI enhances clinical decision-making, leading to improved diagnostic accuracy and speed.
In this blog, you will get to know how is AI helping doctors while accessing the healthcare department. This article explores the various ways in which AI is changing healthcare diagnosis and treatment:
- Advanced Diagnostic Tools: AI helps in developing highly accurate medical imaging algorithms.
- Personalized Treatment Recommendations: Tailoring treatment plans based on individual patient data.
- Benefits and Challenges: Understanding the advantages and addressing the challenges of implementing AI in healthcare.
Artificial intelligence in health care is not just a futuristic concept; it is being adopted at an accelerating pace across the industry. From enhancing operational efficiency to enabling continuous patient monitoring, the growing presence of AI technologies underscores its importance in modern medicine.
Understanding Artificial Intelligence in Healthcare
Medical artificial intelligence (AI) refers to the use of AI technologies in the healthcare industry, covering various functions such as diagnostics and patient care. These technologies rely on large datasets and advanced algorithms to mimic human intelligence in analyzing complex medical information.
Types of AI Technologies in Healthcare
AI technologies commonly found in healthcare include:
- Machine Learning (ML): Uses statistical techniques to enable systems to learn from data and improve over time. Machine learning in healthcare plays a crucial role in predictive analytics and personalized treatment plans.
- Deep Learning: A subset of ML that involves neural networks with multiple layers. Deep learning performs exceptionally well in tasks like image and speech recognition. This makes it highly valuable for medical imaging diagnostics.
Brief History and Promise of AI in Healthcare
The adoption of AI in healthcare started gaining momentum in the early 2000s. Initial applications focused on automating mundane tasks such as scheduling appointments.
Medical care technologies have great potential to transform healthcare artificial intelligence because of their ability to quickly and accurately analyze massive amounts of data. For example, deep learning algorithms can identify abnormalities in medical images that may go unnoticed by human eyes. This leads to earlier detection of diseases and more effective treatments.
Applications of AI in Healthcare
AI in the medical field is transforming disease detection and diagnosis by developing highly accurate medical imaging algorithms. AI medical diagnosis, especially in the fields of radiology and pathology, is gaining attention for its ability to analyze complex medical images with precision.
AI in healthcare examples is changing disease detection and diagnosis including:
- Breast cancer detection: AI algorithms have shown remarkable accuracy in detecting breast cancer through mammograms. These systems can identify subtle patterns and anomalies that might be missed by human eyes.
- Neuroimaging: AI diagnosis is used to analyze MRI and CT scans to detect conditions such as brain tumors, Alzheimer’s disease, and stroke. The technology can quickly process large volumes of data, providing critical insights that aid in timely intervention.
Personalizing Treatment Plans
AI’s capability to process vast amounts of individual patient data enables the personalization of treatment plans. This approach, often referred to as personalized medicine, leverages predictive analytics to tailor interventions based on a patient’s unique genetic makeup, lifestyle, and environmental factors.
How AI is being used to personalize treatment plans:
- Treatment recommendations: The medical AI systems can recommend specific treatments by analyzing patient data from various sources, including electronic health records (EHRs), lab results, and genetic information. For instance, oncology patients can receive customized chemotherapy regimens based on their tumor’s genetic profile.
- Improved outcomes: Personalized treatment plans lead to more effective interventions and better patient outcomes. AI in the healthcare field helps providers make informed decisions that maximize therapeutic efficacy while minimizing adverse effects. This can be done by considering individual variability in the environment.
Continuous Patient Monitoring and Proactive Management
AI-powered chatbots and wearable devices are revolutionizing continuous patient monitoring and proactive health management. These technologies enable remote patient monitoring, ensuring that patients receive timely care without frequent hospital visits.
How AI is transforming continuous patient monitoring and proactive management:
- Wearable sensors: Devices equipped with wearable sensors collect real-time health data such as heart rate, blood pressure, glucose levels, and physical activity. AI algorithms analyze this data to detect abnormalities or trends indicative of potential health issues.
- Patient engagement: AI-driven chatbots provide round-the-clock support by answering patient queries, reminding them about medication schedules, and even guiding them through basic health assessments. This level of interaction enhances patient engagement and adherence to treatment plans.
- Proactive management: Continuous monitoring allows healthcare providers to respond proactively to changes in a patient’s condition. For example, AI in health care can alert physicians if a patient’s vital signs deviate from normal ranges, prompting early intervention before complications arise.
Applications of AI in healthcare extend beyond these examples, continually pushing the boundaries of what’s possible in medical science. The integration of advanced technologies into clinical practice offers promising avenues for improving diagnostic accuracy, personalizing treatments and enhancing overall patient care.
Enhancing Clinical Decision-Making with AI
The integration of AI into clinical decision-making is transforming the landscape of modern healthcare. By leveraging big data analytics and advanced machine learning models, AI in medicine has the potential to improve clinical processes significantly. This transformation is particularly evident in areas like precision medicine and drug discovery.
Precision Medicine
Precision medicine aims to tailor medical treatment to the individual characteristics of each patient. Traditional approaches often rely on generalized treatment plans, but healthcare AI can analyze vast datasets, including genetic information, lifestyle factors, and medical history, to provide more personalized recommendations.
How AI is Advancing Precision Medicine:
- Genomic Analysis: AI algorithms can shift through massive amounts of genomic data to genetic mutations. Deep learning models have been used to predict the likelihood of a patient developing certain types of cancer.
- Predictive Analytics: Machine learning models excel at identifying patterns in patient data that may not be immediately obvious. These patterns can inform predictive analytics, helping healthcare providers foresee potential complications and intervene early. For example, AI systems can predict which patients are at higher risk for conditions like heart disease or diabetes by analyzing their electronic health records (EHRs).
Drug Discovery
The traditional drug discovery process is often lengthy and costly. AI is streamlining this process by speeding up the identification of potential drug candidates and optimizing clinical trials.
How AI is Transforming Drug Discovery:
Compound Screening: Deep learning models can analyze chemical compounds to predict their effectiveness against specific diseases. These models can rapidly screen thousands of compounds, significantly reducing the time required for initial testing phases.
Clinical Trials Optimization: AI can enhance the efficiency of clinical trials by identifying suitable participants based on complex eligibility criteria. Algorithms can also monitor trial progress in real-time, allowing for adjustments that ensure better outcomes.
Challenges in Clinical Decision-Making with AI
Despite its potential benefits, integrating AI into clinical decision-making presents several challenges:
- Data Quality: The accuracy of AI predictions heavily depends on the quality of input data. Inconsistent or incomplete datasets can lead to erroneous conclusions.
- Algorithm Bias: AI models may inadvertently perpetuate existing biases present in training data. Ensuring fairness and accuracy requires ongoing vigilance and refinement of these models.
- Interoperability Issues: Different healthcare systems often use incompatible formats for storing and sharing data. Achieving seamless integration of AI tools across various platforms remains a significant hurdle.
AI technologies are revolutionizing clinical decision-making by offering unprecedented analytical capabilities, enhancing both precision medicine practices and drug discovery efforts. As we continue exploring these advancements, it becomes crucial to address the associated challenges thoughtfully to maximize the benefits while minimizing potential drawbacks.
Benefits and Challenges of AI in Healthcare
Artificial Intelligence in medicine is transforming the healthcare industry, offering new possibilities for improving patient care and operational processes. However, along with its potential benefits, there are also significant challenges and ethical considerations that need to be addressed.
Potential Benefits of AI in Healthcare
1. Enhanced Operational Efficiency
AI technologies streamline numerous administrative and clinical processes in healthcare settings. Tasks like scheduling, billing, and claims processing can be automated using AI systems, which reduces the burden on healthcare staff. This automation allows medical professionals to dedicate more time to patient care.
2. Reduced Costs
Implementing AI in healthcare can lead to significant cost savings. Automated systems reduce the need for manual labor in administrative tasks and improve resource allocation. Early disease detection and accurate diagnosis facilitated by AI can prevent costly treatments for advanced conditions, minimizing overall healthcare expenditures.
3. Improved Patient Outcomes
AI enhances patient outcomes through personalized treatment plans tailored to individual needs. Machine learning algorithms analyze vast datasets, identifying patterns that may not be evident to human clinicians. This capability leads to early diagnosis and intervention, improving recovery rates and reducing complications.
Here are some specific areas where AI is making a difference:
- Medical Imaging: AI excels in analyzing medical images, such as X-rays or MRIs, detecting abnormalities with high accuracy. This aids radiologists in diagnosing conditions like breast cancer early.
- Genomics: AI-powered tools assist in understanding genetic data, enabling precision medicine where treatments are customized based on a patient’s genetic profile.
- Predictive Analytics: AI models predict disease progression and patient responses to treatments, allowing for proactive management and timely interventions.
Key Challenges and Ethical Considerations
1. Data Privacy Concerns
Healthcare data is highly sensitive, making data privacy a paramount concern. The integration of artificial intelligence in healthcare requires access to vast amounts of patient information, raising questions about how this data is stored, shared, and protected. Ensuring compliance with regulations like HIPAA (Health Insurance Portability and Accountability Act) is essential to maintaining trust and safeguarding patient confidentiality.
2. Algorithm Bias
AI systems are only as good as the data they are trained on. If the training data contains biases, these biases can be perpetuated by the algorithms. This bias can lead to disparities in healthcare delivery, affecting diagnosis accuracy and treatment recommendations for different demographic groups. Addressing algorithmic bias involves:
- Diverse Data Sets: Ensuring that training datasets are representative of diverse populations.
- Regular Audits: Conducting ongoing assessments to identify and correct biases.
- Transparency: Maintaining transparency in how algorithms make decisions.
3. Human Oversight
AI systems should augment rather than replace human judgment. The complexity of medical decision-making necessitates human oversight to interpret AI recommendations appropriately. Medical professionals must understand the limitations of AI tools and use them as part of a broader diagnostic or treatment strategy.
4. Interoperability Standards
The seamless integration of various AI systems into existing healthcare infrastructures is another challenge. Different systems need standardized protocols to communicate effectively with each other, ensuring that information flows smoothly across platforms without data loss or misinterpretation.
Balancing Benefits with Challenges
While the benefits of AI in healthcare are substantial—improved efficiency, reduced costs, better patient outcomes—the challenges cannot be ignored. Achieving a balance involves:
- Robust Data Security Measures: Implementing strong encryption methods and ensuring compliance with regulatory standards.
- Ethical Frameworks: Developing guidelines that address ethical concerns related to bias, transparency, and accountability.
- Continuous Monitoring: Regularly updating algorithms based on new data and feedback from clinical use.
The Future of AI in Healthcare
Emerging Trends and Future Possibilities
AI is set to play a crucial role in mental health support. Innovations like AI-driven chatbots and virtual therapists are offering immediate, accessible mental health assistance, breaking down barriers to care. These tools use natural language processing (NLP) to provide therapeutic conversations, mood tracking, and even crisis intervention.
Example: Woebot is an AI-powered chatbot providing cognitive-behavioral therapy techniques to users, offering real-time mental health support.
Another significant area is improving healthcare accessibility in underserved regions. AI can bridge gaps where medical professionals are scarce:
- Telemedicine Platforms: Enhanced by AI, these platforms can diagnose and recommend treatments remotely.
- Wearable Devices: Equipped with AI, they can monitor vital signs and alert healthcare providers in case of abnormalities, ensuring timely interventions.
Responsible and Ethical Implementation
Balancing innovation with patient safety and privacy remains crucial. Ensuring ethical implementation involves addressing several key areas:
- Data Privacy: Protecting patient information through robust encryption methods and compliance with regulations like GDPR.
- Algorithmic Bias: Developing unbiased algorithms by training them on diverse datasets to avoid disparities in healthcare delivery.
- Transparency: Making AI decision-making processes understandable to clinicians and patients alike.
Emerging trends suggest the future of AI in healthcare will also see advancements in precision medicine, genomics, and predictive analytics. By leveraging big data, AI can anticipate disease outbreaks, personalize treatments based on genetic profiles, and optimize resource allocation within healthcare facilities.
The journey ahead promises transformative changes; however, it demands a responsible approach that prioritizes ethical considerations alongside technological advancements.
Conclusion
The healthcare industry needs to embrace the potential of AI revolutionizing healthcare diagnosis and treatment to drive positive change. AI offers great opportunities for improved efficiency, accuracy, and patient outcomes.
Healthcare professionals should make an effort to stay updated on the latest advancements in AI technology. By using these tools responsibly in their practice, they can ensure that patients receive the most accurate diagnoses and personalized treatments possible.
Ongoing research and development are necessary to overcome the limitations of AI in healthcare. This includes addressing ethical concerns, protecting data privacy, and minimizing algorithmic bias. These are crucial for building trust and maximizing the benefits of AI applications.
Integrating artificial intelligence diagnosis in the medical field not only enhances clinical decision-making but also frees up valuable time for providers to dedicate more attention to patient care. Being knowledgeable and proactive about AI advancements is a critical step toward creating a more efficient and effective healthcare system.
Encourage a commitment to continuous learning and responsible implementation of AI technologies. The future of healthcare relies on embracing innovation while safeguarding patient safety and privacy.