Role of AI in Banking
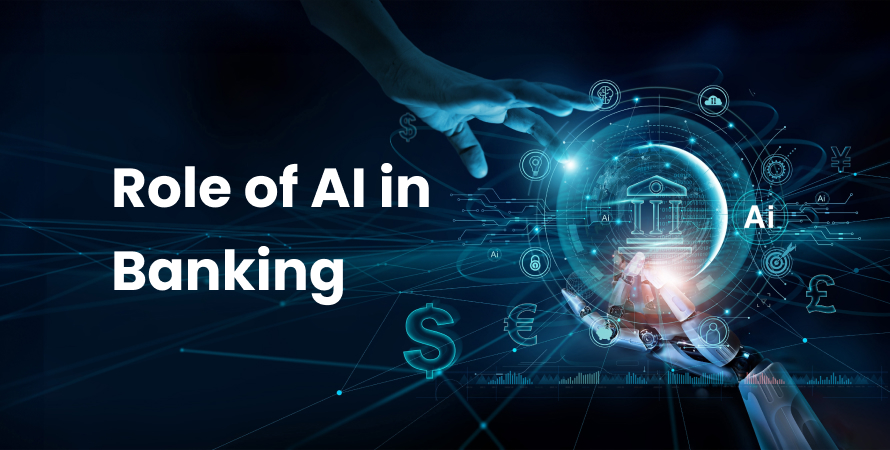
Artificial Intelligence (AI) is significantly reshaping the banking sector, driving substantial changes in how financial institutions operate. The Role of AI in Banking extends far beyond a mere technological trend; it represents a transformative force capable of revolutionizing the industry.
Here, in this blog, you will learn about the role of AI in banking/financial services.
Influence on the Banking Sector
Artificial intelligence in banking sector has changed the banking sector by enhancing efficiency, security, and customer experience.
- Operational Efficiency: AI technologies allow banks to automate routine tasks, reducing operational costs and minimizing human error.
- Customer Experience: Enhanced capabilities such as AI-powered chatbots provide 24/7 customer support, delivering personalized and efficient service.
- Risk Mitigation: Advanced algorithms enable real-time fraud detection and improved credit scoring, safeguarding both banks and their customers.
AI in financial services enhances cyber security by detecting fraudulent activities in real-time. Comprehending how financial AI enhances banking services is crucial for stakeholders. Recognizing its potential helps in:
- Optimizing Resources: Efficiently allocating resources and improving decision-making processes.
- Personalization: Tailoring services to meet individual customer needs based on data-driven insights.
- Compliance and Security: Navigating complex regulatory environments and ensuring robust cybersecurity measures.
Key Takeaway
AI in banking and finance is not just a fleeting innovation. It holds the promise to drive efficiency, improve customer experiences, and mitigate risks. However, to fully harness its potential, banks must adeptly navigate the accompanying challenges.
The Evolution of Banking with Artificial Intelligence
Historical Context and Key Milestones
The banking sector has a rich history of technological adoption that has consistently revolutionized its operations. Key milestones include:
- Introduction of ATMs: Automated Teller Machines (ATMs) in the late 1960s provided customers with unprecedented access to their funds outside bank hours.
- Online Banking: The 1990s saw the rise of online banking, enabling customers to manage accounts, transfer money, and pay bills from the comfort of their homes.
AI as a Transformative Force
Artificial Intelligence in financial services represents a significant leap forward in banking evolution. By processing vast amounts of data quickly and accurately, AI shifts both operations and customer engagement to new heights. Examples include:
- Data Processing: AI can analyze large datasets in real time, allowing for faster decision-making.
- Customer Predictions: Predictive analytics enable banks to anticipate customer needs and behaviors more accurately.
Key Areas of Impact
AI’s influence spans multiple facets of banking:
- Fraud Detection: Real-time analysis of transaction patterns helps identify fraudulent activities promptly.
- Customer Support: AI-powered chatbots provide 24/7 support across various channels, enhancing customer service.
- Credit Scoring: Machine learning algorithms deliver more accurate credit assessments by considering diverse data points beyond traditional credit history.
- Process Automation: Robotic Process Automation (RPA) handles routine tasks such as data entry, reducing operational costs and human error.
- Market Forecasting: Predictive models trained on historical and external data help banks anticipate market trends and optimize resource allocation.
- Personalization: Advanced segmentation techniques allow for targeted service offerings based on unique customer needs and preferences.
- Regulatory Compliance: AI assists in navigating complex regulatory landscapes by ensuring efficient compliance with legal standards.
- Cybersecurity: Enhanced monitoring systems powered by AI detect vulnerabilities and defend against cyber threats effectively.
- Operational Efficiency: Streamlined operations result from faster transaction processing and improved overall service delivery through AI integration.
- Data Management: Quality data policies are crucial for reliable AI applications, ensuring accurate insights and decisions.
Banking’s evolution with artificial intelligence is not just about adopting new technology; it’s about reshaping the entire landscape to become more efficient, secure, and customer-centric.
Fraud Detection and Prevention
AI investment banking plays a crucial role in fraud detection by analyzing transaction patterns to identify anomalies in real time. Through sophisticated anomaly detection algorithms, AI can scrutinize vast volumes of transactions, flagging any irregular activities that diverge from established norms.
Key Benefits
- Rapid Response: Automated alerts enable banks to respond swiftly to potential threats.
- Enhanced Security: Continuous monitoring and instant investigations help mitigate fraudulent activities before they escalate.
Enhanced Customer Support with Chatbots
AI-powered chatbots and virtual assistants are transforming the customer experience in banking by providing 24/7 support across various channels such as website chat and messaging apps. This constant availability ensures that customers can get help whenever they need it, making their banking experience more convenient and satisfying.
Personalization Through Data Analysis
Chatbots use data analysis to understand past interactions and preferences. This allows them to provide personalized responses to each customer. This customization goes beyond just addressing queries; it also includes suggesting products or services that match the customer’s previous behavior and requirements, resulting in more meaningful and interesting conversations.
Impact on Customer Satisfaction
The implementation of chatbots has a significant positive effect on important customer satisfaction metrics:
- Resolution Time: Automated systems handle inquiries swiftly, drastically reducing wait times.
- Overall Experience Ratings: Personalized, immediate responses contribute to higher overall satisfaction scores.
Banks that utilize AI-powered chatbots not only streamline their customer service processes but also create positive experiences for their clients.
Improving Credit Scoring Accuracy using Machine Learning Algorithms
Machine learning algorithms enhance predictive power by considering a wider range of variables beyond traditional credit history. For instance, non-traditional data sources such as social media activity and spending habits provide a more comprehensive view of an individual’s financial behavior. This allows banks to make more informed lending decisions.
Comparison with Conventional Methods
- Traditional Credit Scoring: This relies heavily on historical credit data, which may not fully capture a borrower’s current financial situation.
- AI-Powered Techniques: Utilize advanced algorithms like gradient boosting and neural networks to analyze complex datasets. These models are often evaluated using metrics such as the AUC-ROC curve, demonstrating higher accuracy in predicting default risks.
Implications for Lending Practices
- Inclusive Access to Credit: Individuals who lack extensive credit histories, such as young adults or immigrants, can gain access to credit. Financial artificial intelligence models assess their risk based on diverse data points without compromising default risk management standards.
- Enhanced Risk Assessment: By incorporating a broader range of data, banking and AI achieve not only a higher degree of accuracy but also more reliable risk assessments, ensuring better financial health for both lenders and borrowers.
Streamlining Banking Operations through Process Automation
Process automation is transforming how banks manage their everyday tasks. A key technology driving this change is Robotic Process Automation (RPA), which can automate repetitive tasks such as:
- Entering data from customer forms into backend systems.
- Conducting compliance checks and generating reports.
- Reconciling accounts.
- Onboarding new customers.
- Transform credit review complaints.
Implementing RPA not only speeds up these processes but also significantly reduces operational costs. Banks can achieve high accuracy levels due to decreased human error rates, leading to operational efficiency improvements.
By leveraging RPA, banks are poised to reallocate human resources to more strategic roles, fostering innovation and enhancing overall productivity.
Using Predictive Analytics to Forecast Market Trends
Predictive analytics applications are changing the way banks predict market trends and customer behaviors. One notable use case involves a bank using machine learning models trained on historical transaction data, combined with external economic indicators. This approach enabled the bank to accurately predict changes in demand for specific financial products and services.
Benefits
- Proactive Resource Allocation: With accurate forecasts, banks can optimize their marketing spend based on anticipated sales volumes, ensuring resources are allocated where they will yield the highest returns.
- Enhanced Decision-Making: Predictive insights allow banks to make informed decisions about product development and customer engagement strategies.
By integrating predictive analytics, banks can stay ahead of market trends, offering tailored solutions that meet evolving customer needs while efficiently managing resources.
Personalizing Banking Experiences at Scale
Personalized services delivery in banking uses advanced segmentation techniques powered by clustering algorithms. These algorithms help banks identify distinct customer groups with unique needs and interests, allowing for targeted service offerings. For example, personalized investment banking artificial intelligence portfolios can be tailored to specific customer segments based on their financial goals and risk tolerance.
Challenges in Implementation
Some challenges during implementation include:
- Ensuring seamless integration between the front-end interfaces used by customers and the back-end systems responsible for executing these tailored transactions efficiently.
- Maintaining robust infrastructure and sophisticated data management practices to ensure real-time accuracy and responsiveness.
Benefits of Personalization
By addressing these challenges, banks can deliver highly customized experiences that enhance customer satisfaction and loyalty.
Navigating Regulatory Compliance Challenges with Technology Solutions
Regulatory compliance automation tools play a crucial role in helping banks meet strict regulatory requirements. Key regulations that affect banks worldwide include:
- Anti-Money Laundering (AML) laws
- Know Your Customer (KYC) guidelines
- General Data Protection Regulation (GDPR)
Banks must consistently fulfill these compliance obligations within specified time frames set by regulators.
To tackle these challenges, banks use AI-powered tools that automate compliance processes, ensuring accuracy and timely reporting. These technologies monitor transactions in real-time, flagging suspicious activities that may indicate money laundering or fraud. Additionally, AI systems help maintain comprehensive records that are easily retrievable for audits.
Implementing such solutions not only reduces the risks of non-compliance but also minimizes the manual effort required for regulatory tasks, allowing staff to concentrate on more strategic activities.
Strengthening Cybersecurity Posture against Evolving Threats
Enhancing cybersecurity strategies is essential in the banking industry because cyber threats are constantly changing. Banks need to use a multi-layered approach to defense that includes both preventive and proactive measures:
Preventive Measures
Traditional defenses such as firewalls and encryption serve as the initial line of defense against potential breaches.
Proactive Monitoring
Using AI-based intrusion detection systems (IDS) improves real-time monitoring capabilities. These systems examine network traffic for unusual patterns that may indicate potential breaches, allowing for quick action against emerging threats.
By combining these strategies, banks can significantly strengthen their cybersecurity posture, ensuring better protection of sensitive financial data and maintaining customer trust.
Challenges and Considerations in Implementing AI in Banking
Ethical Considerations
Artificial Intelligence (AI) introduces several ethical dilemmas within the banking sector. One pressing concern is the potential for algorithmic bias, which can lead to unfair treatment of certain customer segments. Algorithms trained on historical data may inadvertently perpetuate existing inequalities, resulting in biased decision-making processes. For instance, automated loan approvals might favor applicants from specific demographic backgrounds while disadvantaging others.
Data Privacy
The integration of AI in finance industry necessitates access to vast amounts of personal and financial data. Protecting this data becomes paramount to maintain consumer trust. Banks must ensure robust data privacy measures are in place to prevent unauthorized access and breaches. This includes complying with stringent regulations such as the General Data Protection Regulation (GDPR) and the California Consumer Privacy Act (CCPA).
Explainable AI
Transparency around AI decision-making is critical, especially when it comes to high-stakes decisions like AI loan approvals or credit scoring. Explainable AI (XAI) aims to make the reasoning behind automated outcomes understandable to both customers and regulatory bodies. This transparency helps ensure fairness and builds trust by allowing individuals to see how decisions impacting their financial well-being are made.
Key Challenges:
- Bias in Algorithms: Ensuring AI models are trained on diverse datasets to minimize inherent biases.
- Maintaining Trust: Balancing efficiency with transparency, especially for critical roles traditionally performed by humans.
- Data Security: Implementing robust safeguards to protect sensitive information from cyber threats and unauthorized use.
- Regulatory Compliance: Keeping up with evolving legal standards related to AI and data privacy.
Banks must navigate these challenges carefully to harness the full potential of AI while maintaining consumer confidence and adhering to ethical standards.
Conclusion
The integration of Artificial Intelligence in the banking sector signifies a revolutionary shift towards enhanced efficiency, personalized customer experiences, and robust risk management. As banks continue to leverage AI technologies for fraud detection, customer support, credit scoring, process automation, and cybersecurity. They must also navigate the ethical and regulatory challenges associated with data privacy and algorithmic transparency.